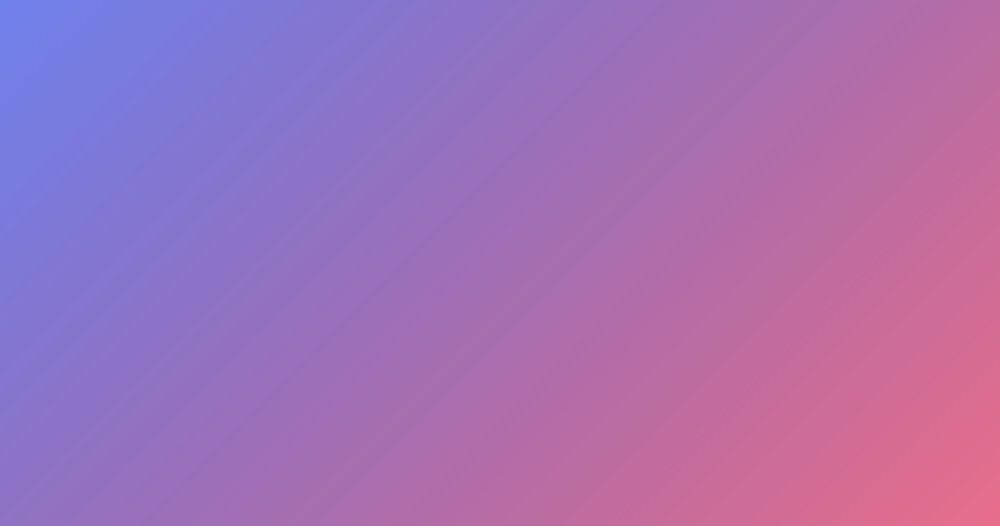
Why Ignoring Deepseek Will Cost You Sales
free deepseek additionally raises questions on Washington's efforts to comprise Beijing's push for tech supremacy, provided that certainly one of its key restrictions has been a ban on the export of superior chips to China. However, we do not need to rearrange experts since every GPU only hosts one skilled. Finally, we're exploring a dynamic redundancy strategy for specialists, the place every GPU hosts more consultants (e.g., Sixteen consultants), but solely 9 shall be activated throughout every inference step. The excessive-load consultants are detected based on statistics collected during the web deployment and are adjusted periodically (e.g., every 10 minutes). Just like prefilling, we periodically decide the set of redundant experts in a sure interval, based mostly on the statistical professional load from our online service. D is ready to 1, i.e., in addition to the exact subsequent token, every token will predict one extra token. On FRAMES, a benchmark requiring question-answering over 100k token contexts, DeepSeek-V3 carefully trails GPT-4o while outperforming all other fashions by a major margin. In lengthy-context understanding benchmarks similar to DROP, LongBench v2, and FRAMES, DeepSeek-V3 continues to exhibit its position as a prime-tier model. LongBench v2: Towards deeper understanding and reasoning on practical lengthy-context multitasks.
Early reasoning steps would operate in an unlimited but coarse-grained space. The put up-coaching also makes a hit in distilling the reasoning capability from the DeepSeek-R1 series of fashions. Qwen and DeepSeek are two consultant mannequin collection with sturdy support for both Chinese and English. Secondly, though our deployment strategy for DeepSeek-V3 has achieved an end-to-finish technology speed of more than two times that of DeepSeek-V2, there nonetheless stays potential for further enhancement. Based on our evaluation, the acceptance charge of the second token prediction ranges between 85% and 90% across numerous era subjects, demonstrating consistent reliability. Overall, the CodeUpdateArena benchmark represents an necessary contribution to the continuing efforts to enhance the code generation capabilities of large language fashions and make them extra robust to the evolving nature of software program improvement. Additionally, we will strive to interrupt by the architectural limitations of Transformer, thereby pushing the boundaries of its modeling capabilities.
As the system's capabilities are additional developed and its limitations are addressed, it could grow to be a robust device within the fingers of researchers and downside-solvers, serving to them deal with more and more difficult problems more efficiently. I worked carefully with MCTS for a number of years while at DeepMind, and there are various implementation details that I feel researchers (resembling DeepSeek) are both getting incorrect or not discussing clearly. In January 2025, Western researchers were able to trick DeepSeek into giving certain solutions to a few of these matters by requesting in its reply to swap certain letters for similar-wanting numbers. For instance, the model refuses to reply questions about the 1989 Tiananmen Square massacre, persecution of Uyghurs, deepseek comparisons between Xi Jinping and Winnie the Pooh, and human rights in China. For instance, I tasked Sonnet with writing an AST parser for Jsonnet, and it was in a position to take action with minimal further help.
For example, the synthetic nature of the API updates could not absolutely seize the complexities of actual-world code library modifications. It is a prepared-made Copilot that you could integrate with your software or any code you can access (OSS). To address this inefficiency, we recommend that future chips combine FP8 cast and TMA (Tensor Memory Accelerator) access right into a single fused operation, so quantization will be accomplished through the switch of activations from world memory to shared memory, avoiding frequent reminiscence reads and writes. Higher FP8 GEMM Accumulation Precision in Tensor Cores. Although the dequantization overhead is considerably mitigated mixed with our exact FP32 accumulation strategy, the frequent data movements between Tensor Cores and CUDA cores nonetheless restrict the computational effectivity. Specifically, while the R1-generated data demonstrates strong accuracy, it suffers from issues such as overthinking, poor formatting, and excessive length. This method ensures that the ultimate coaching data retains the strengths of DeepSeek-R1 whereas producing responses that are concise and efficient.
In case you loved this information and you wish to receive more info regarding ديب سيك please visit the web site.
Reviews