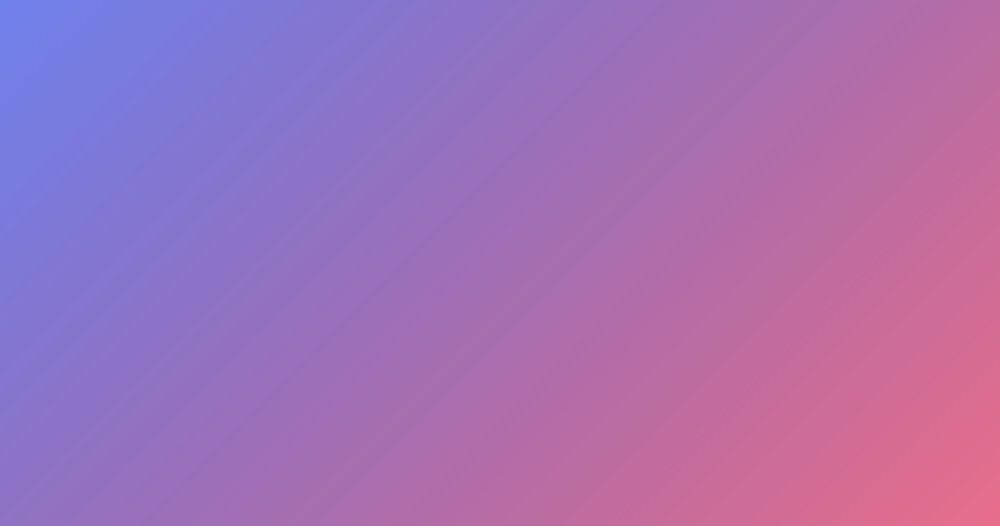
By no means Endure From Deepseek Once more
Specifically, DeepSeek introduced Multi Latent Attention designed for environment friendly inference with KV-cache compression. In February 2024, DeepSeek introduced a specialized mannequin, DeepSeekMath, with 7B parameters. Much like DeepSeek-V2 (DeepSeek-AI, 2024c), we undertake Group Relative Policy Optimization (GRPO) (Shao et al., 2024), which foregoes the critic mannequin that is typically with the same measurement as the policy mannequin, and estimates the baseline from group scores instead. In addition to plain benchmarks, we additionally consider our models on open-ended era duties utilizing LLMs as judges, with the results proven in Table 7. Specifically, we adhere to the original configurations of AlpacaEval 2.Zero (Dubois et al., 2024) and Arena-Hard (Li et al., 2024a), which leverage GPT-4-Turbo-1106 as judges for pairwise comparisons. You can use GGUF fashions from Python utilizing the llama-cpp-python or ctransformers libraries. In DeepSeek you simply have two - DeepSeek-V3 is the default and if you'd like to use its superior reasoning mannequin you must tap or click on the 'DeepThink (R1)' button before coming into your prompt. On C-Eval, a consultant benchmark for Chinese instructional information analysis, and CLUEWSC (Chinese Winograd Schema Challenge), DeepSeek-V3 and Qwen2.5-72B exhibit comparable efficiency levels, indicating that each fashions are properly-optimized for difficult Chinese-language reasoning and instructional tasks.
The paper attributes the sturdy mathematical reasoning capabilities of DeepSeekMath 7B to 2 key components: the intensive math-related information used for pre-coaching and the introduction of the GRPO optimization approach. This underscores the strong capabilities of DeepSeek-V3, especially in dealing with complicated prompts, including coding and debugging tasks. This demonstrates the robust capability of DeepSeek-V3 in handling extraordinarily long-context tasks. This demonstrates its outstanding proficiency in writing duties and handling easy query-answering situations. MMLU is a broadly acknowledged benchmark designed to assess the efficiency of large language models, across diverse information domains and duties. This is extra difficult than updating an LLM's knowledge about general info, because the model should cause in regards to the semantics of the modified function reasonably than simply reproducing its syntax. I lately had the opportunity to make use of DeepSeek, and I need to say, it has completely transformed the way in which I method knowledge evaluation and decision-making. This strategy not solely aligns the mannequin extra intently with human preferences but additionally enhances performance on benchmarks, particularly in situations the place available SFT data are limited. "The sort of data collected by AutoRT tends to be extremely various, resulting in fewer samples per activity and plenty of selection in scenes and object configurations," Google writes.
"The DeepSeek mannequin rollout is leading investors to query the lead that US companies have and how a lot is being spent and whether or not that spending will result in profits (or overspending)," stated Keith Lerner, analyst at Truist. AI will exchange/ won’t substitute my coding expertise. That is coming natively to Blackwell GPUs, which can be banned in China, but DeepSeek constructed it themselves! Each submitted answer was allocated either a P100 GPU or 2xT4 GPUs, with up to 9 hours to solve the 50 issues. On the more difficult FIMO benchmark, DeepSeek-Prover solved 4 out of 148 issues with 100 samples, whereas GPT-four solved none. For mathematical assessments, AIME and CNMO 2024 are evaluated with a temperature of 0.7, and the results are averaged over sixteen runs, while MATH-500 employs greedy decoding. We utilize the Zero-Eval immediate format (Lin, 2024) for MMLU-Redux in a zero-shot setting. For example, certain math issues have deterministic results, and we require the model to offer the ultimate answer within a chosen format (e.g., in a field), allowing us to use guidelines to verify the correctness. On the instruction-following benchmark, DeepSeek-V3 considerably outperforms its predecessor, DeepSeek-V2-sequence, highlighting its improved ability to understand and adhere to person-defined format constraints.
Specifically, on AIME, MATH-500, and CNMO 2024, DeepSeek-V3 outperforms the second-greatest model, Qwen2.5 72B, by approximately 10% in absolute scores, which is a considerable margin for such difficult benchmarks. In engineering tasks, deepseek ai china-V3 trails behind Claude-Sonnet-3.5-1022 however significantly outperforms open-source fashions. By providing entry to its robust capabilities, DeepSeek-V3 can drive innovation and improvement in areas akin to software engineering and algorithm growth, empowering developers and researchers to push the boundaries of what open-supply fashions can achieve in coding tasks. Conversely, for questions without a definitive ground-fact, resembling these involving artistic writing, the reward mannequin is tasked with offering suggestions based mostly on the question and the corresponding answer as inputs. For questions that can be validated using specific rules, we undertake a rule-based reward system to find out the suggestions. Similarly, for LeetCode problems, we are able to make the most of a compiler to generate feedback based on take a look at instances. This success could be attributed to its advanced data distillation approach, which successfully enhances its code generation and downside-solving capabilities in algorithm-focused tasks. This achievement significantly bridges the performance gap between open-supply and closed-source fashions, setting a new normal for what open-supply fashions can accomplish in difficult domains.
Reviews