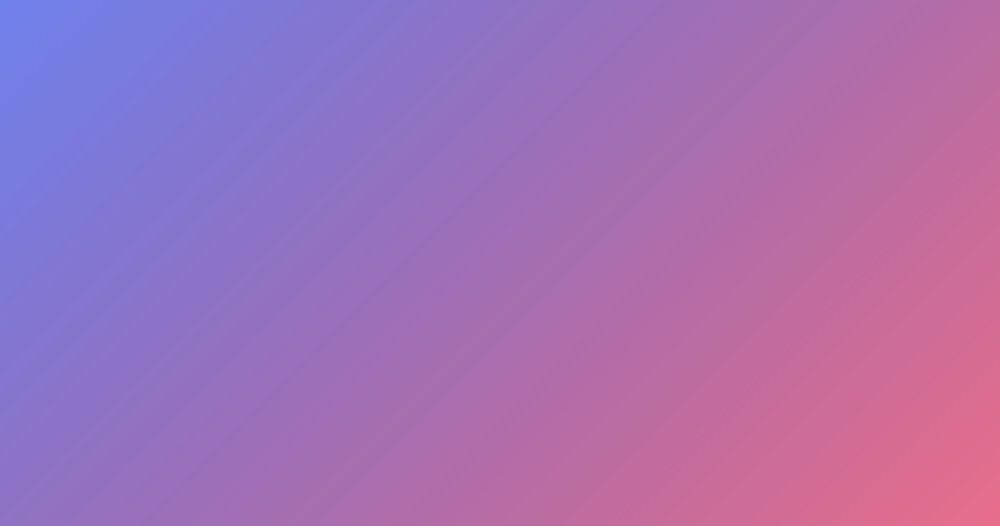
DeepSeek-Coder-V2: Breaking the Barrier of Closed-Source Models In Code Intelligence
But the DeepSeek development might point to a path for the Chinese to catch up more quickly than beforehand thought. As for Chinese benchmarks, aside from CMMLU, a Chinese multi-subject a number of-choice activity, DeepSeek-V3-Base also shows better efficiency than Qwen2.5 72B. (3) Compared with LLaMA-3.1 405B Base, the largest open-source mannequin with 11 times the activated parameters, DeepSeek-V3-Base also exhibits much better efficiency on multilingual, code, and math benchmarks. 2) Compared with Qwen2.5 72B Base, the state-of-the-art Chinese open-supply mannequin, with only half of the activated parameters, DeepSeek-V3-Base additionally demonstrates outstanding advantages, particularly on English, multilingual, code, and math benchmarks. DeepSeek V3 and DeepSeek V2.5 use a Mixture of Experts (MoE) structure, while Qwen2.5 and Llama3.1 use a Dense architecture. We conduct complete evaluations of our chat mannequin against a number of strong baselines, together with DeepSeek-V2-0506, DeepSeek-V2.5-0905, Qwen2.5 72B Instruct, LLaMA-3.1 405B Instruct, Claude-Sonnet-3.5-1022, and GPT-4o-0513. The deepseek ai china Chat V3 model has a high rating on aider’s code enhancing benchmark. Each mannequin is pre-trained on challenge-stage code corpus by using a window size of 16K and a additional fill-in-the-blank activity, to help challenge-degree code completion and infilling. 1) Compared with deepseek ai-V2-Base, because of the enhancements in our model architecture, the scale-up of the mannequin dimension and coaching tokens, and the enhancement of information quality, DeepSeek-V3-Base achieves significantly higher performance as expected.
POSTSUPERSCRIPT in 4.3T tokens, following a cosine decay curve. 0.Three for the primary 10T tokens, and to 0.1 for the remaining 4.8T tokens. At the large scale, we practice a baseline MoE model comprising 228.7B total parameters on 578B tokens. Pretty good: They practice two types of mannequin, a 7B and a 67B, then they examine performance with the 7B and 70B LLaMa2 fashions from Facebook. From a extra detailed perspective, we evaluate DeepSeek-V3-Base with the other open-supply base fashions individually. Compared with the sequence-clever auxiliary loss, batch-wise balancing imposes a extra flexible constraint, as it does not implement in-domain stability on every sequence. The important thing distinction between auxiliary-loss-free balancing and sequence-clever auxiliary loss lies of their balancing scope: batch-clever versus sequence-smart. As illustrated in Figure 9, we observe that the auxiliary-loss-free mannequin demonstrates greater expert specialization patterns as anticipated. From the table, we are able to observe that the auxiliary-loss-free technique consistently achieves higher model performance on many of the analysis benchmarks.
To be particular, we validate the MTP technique on top of two baseline fashions throughout completely different scales. Note that during inference, we straight discard the MTP module, so the inference costs of the compared models are precisely the identical. In addition, although the batch-clever load balancing strategies present consistent performance advantages, they also face two potential challenges in effectivity: (1) load imbalance inside certain sequences or small batches, and (2) domain-shift-induced load imbalance throughout inference. For the second problem, we also design and implement an efficient inference framework with redundant skilled deployment, as described in Section 3.4, to overcome it. The training course of entails producing two distinct types of SFT samples for each instance: the primary couples the problem with its original response within the format of , while the second incorporates a system immediate alongside the problem and the R1 response in the format of . For instance, certain math problems have deterministic results, and we require the mannequin to provide the ultimate answer within a designated format (e.g., in a box), permitting us to apply rules to verify the correctness. We utilize the Zero-Eval prompt format (Lin, 2024) for MMLU-Redux in a zero-shot setting. The system immediate is meticulously designed to incorporate directions that guide the model towards producing responses enriched with mechanisms for reflection and verification.
For non-reasoning data, comparable to inventive writing, function-play, and simple question answering, we make the most of DeepSeek-V2.5 to generate responses and enlist human annotators to confirm the accuracy and correctness of the data. Through the RL part, the mannequin leverages high-temperature sampling to generate responses that combine patterns from each the R1-generated and unique knowledge, even within the absence of explicit system prompts. Specifically, whereas the R1-generated knowledge demonstrates robust accuracy, it suffers from points reminiscent of overthinking, poor formatting, and extreme size. Our goal is to balance the excessive accuracy of R1-generated reasoning data and the clarity and conciseness of frequently formatted reasoning knowledge. Upon completing the RL training part, we implement rejection sampling to curate high-high quality SFT information for the final model, where the skilled models are used as data technology sources. DeepSeek's flagship mannequin, DeepSeek-R1, is designed to generate human-like text, enabling context-conscious dialogues appropriate for functions corresponding to chatbots and customer support platforms. Because HumanEval/MBPP is just too simple (basically no libraries), additionally they test with DS-1000.
Reviews