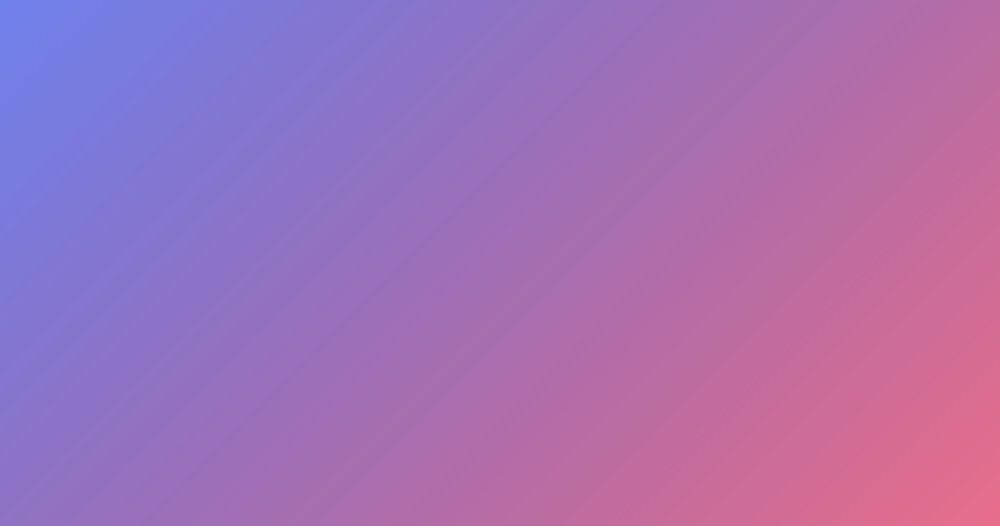
Find Out Now, What Should you Do For Quick Deepseek?
Like several laboratory, DeepSeek absolutely has different experimental items going in the background too. A/H100s, line items resembling electricity end up costing over $10M per year. This year now we have seen important enhancements on the frontier in capabilities in addition to a brand new scaling paradigm. When you have a sweet tooth for this kind of music (e.g. enjoy Pavement or Pixies), it could also be worth checking out the rest of this album, Mindful Chaos. Looks like we might see a reshape of AI tech in the approaching year. This looks like 1000s of runs at a really small size, seemingly 1B-7B, to intermediate data quantities (wherever from Chinchilla optimal to 1T tokens). Strong effort in constructing pretraining information from Github from scratch, with repository-degree samples. Get the benchmark right here: BALROG (balrog-ai, GitHub). Hence, I ended up sticking to Ollama to get something running (for now). "How can humans get away with simply 10 bits/s?
The eye is All You Need paper introduced multi-head attention, which could be considered: "multi-head attention allows the mannequin to jointly attend to info from completely different representation subspaces at completely different positions. Then, the latent part is what DeepSeek launched for the DeepSeek V2 paper, where the model saves on reminiscence utilization of the KV cache by using a low rank projection of the attention heads (at the potential cost of modeling performance). On the TruthfulQA benchmark, InstructGPT generates truthful and informative solutions about twice as often as GPT-3 During RLHF fine-tuning, we observe efficiency regressions in comparison with GPT-3 We can vastly cut back the performance regressions on these datasets by mixing PPO updates with updates that enhance the log likelihood of the pretraining distribution (PPO-ptx), without compromising labeler preference scores. Overall, ChatGPT gave the very best answers - but we’re still impressed by the level of "thoughtfulness" that Chinese chatbots show. This needs to be interesting to any builders working in enterprises which have knowledge privacy and sharing issues, but still want to improve their developer productivity with domestically working models. This does not account for different initiatives they used as ingredients for deepseek ai V3, similar to DeepSeek r1 lite, which was used for synthetic knowledge.
When you employ Continue, you mechanically generate information on how you construct software. Now that we know they exist, many groups will build what OpenAI did with 1/10th the price. It is a state of affairs OpenAI explicitly needs to keep away from - it’s higher for them to iterate quickly on new models like o3. DeepSeekMath 7B's efficiency, which approaches that of state-of-the-art fashions like Gemini-Ultra and GPT-4, demonstrates the numerous potential of this approach and its broader implications for fields that depend on superior mathematical abilities. Others demonstrated easy but clear examples of superior Rust utilization, like Mistral with its recursive approach or Stable Code with parallel processing. I’d guess the latter, since code environments aren’t that simple to setup. It excels in areas which are historically difficult for AI, like advanced mathematics and code generation. GPT-2, while pretty early, confirmed early indicators of potential in code generation and developer productivity improvement. That is one of those things which is both a tech demo and also an necessary signal of things to return - in the future, we’re going to bottle up many different parts of the world into representations realized by a neural web, then allow these items to come alive inside neural nets for countless generation and recycling.
For one example, consider evaluating how the DeepSeek V3 paper has 139 technical authors. Their style, too, is one of preserved adolescence (maybe not uncommon in China, with awareness, reflection, rebellion, and even romance put off by Gaokao), contemporary but not totally innocent. That is coming natively to Blackwell GPUs, which might be banned in China, but DeepSeek built it themselves! The prices to prepare models will proceed to fall with open weight fashions, especially when accompanied by detailed technical stories, but the pace of diffusion is bottlenecked by the necessity for challenging reverse engineering / reproduction efforts. Common practice in language modeling laboratories is to make use of scaling laws to de-risk ideas for pretraining, so that you just spend little or no time coaching at the biggest sizes that do not lead to working fashions. I’ll be sharing more soon on easy methods to interpret the stability of energy in open weight language models between the U.S. There’s a lot more commentary on the fashions on-line if you’re in search of it. The success right here is that they’re relevant among American expertise corporations spending what is approaching or surpassing $10B per yr on AI fashions.
Reviews