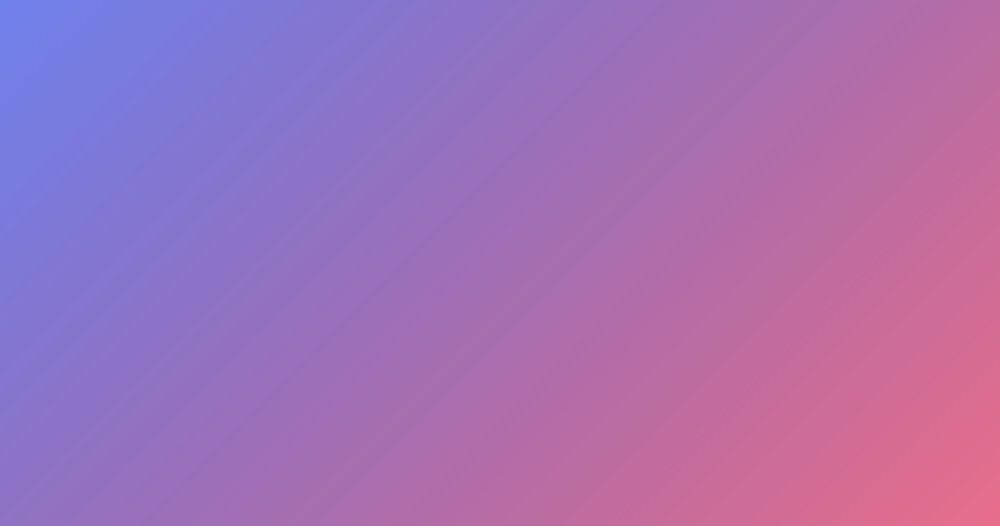
What's so Valuable About It?
This post revisits the technical details of DeepSeek V3, but focuses on how greatest to view the cost of training models on the frontier of AI and how these prices may be changing. As did Meta’s update to Llama 3.3 mannequin, which is a better put up practice of the 3.1 base fashions. It’s onerous to filter it out at pretraining, especially if it makes the model higher (so you might want to turn a blind eye to it). For example, a 175 billion parameter mannequin that requires 512 GB - 1 TB of RAM in FP32 may potentially be diminished to 256 GB - 512 GB of RAM by utilizing FP16. For instance, RL on reasoning could improve over extra coaching steps. In two more days, the run would be complete. The two V2-Lite models were smaller, and skilled equally, though DeepSeek-V2-Lite-Chat only underwent SFT, not RL. The fashions tested didn't produce "copy and paste" code, however they did produce workable code that offered a shortcut to the langchain API. As with tech depth in code, talent is comparable. I’ve seen quite a bit about how the talent evolves at different levels of it. For the final week, I’ve been utilizing DeepSeek V3 as my each day driver for regular chat tasks.
It’s a really capable mannequin, but not one that sparks as much joy when using it like Claude or with tremendous polished apps like ChatGPT, so I don’t anticipate to maintain using it long run. Model quantization enables one to scale back the memory footprint, and improve inference speed - with a tradeoff against the accuracy. In the course of the submit-training stage, we distill the reasoning capability from the DeepSeek-R1 sequence of models, and ديب سيك meanwhile carefully maintain the balance between mannequin accuracy and era size. First, Cohere’s new model has no positional encoding in its world attention layers. Multi-head latent attention (MLA)2 to attenuate the memory usage of consideration operators whereas sustaining modeling performance. We profile the peak memory utilization of inference for 7B and 67B models at totally different batch size and sequence length settings. In exams across all the environments, one of the best models (gpt-4o and claude-3.5-sonnet) get 32.34% and 29.98% respectively. We tried. We had some ideas that we wanted people to depart these companies and begin and it’s really laborious to get them out of it. They have, by far, the most effective model, by far, the very best access to capital and GPUs, and they have one of the best folks.
You've a lot of people already there. The deepseek ai LLM 7B/67B Base and DeepSeek LLM 7B/67B Chat versions have been made open source, aiming to assist analysis efforts in the sector. Overall, the CodeUpdateArena benchmark represents an important contribution to the continued efforts to enhance the code generation capabilities of giant language models and make them extra robust to the evolving nature of software program development. Because it will change by nature of the work that they’re doing. And maybe more OpenAI founders will pop up. I don’t actually see a whole lot of founders leaving OpenAI to start out something new because I feel the consensus within the company is that they are by far one of the best. For Chinese companies which are feeling the pressure of substantial chip export controls, it can't be seen as notably surprising to have the angle be "Wow we will do manner more than you with much less." I’d probably do the same in their shoes, it is far more motivating than "my cluster is bigger than yours." This goes to say that we'd like to know how necessary the narrative of compute numbers is to their reporting. Among the many universal and loud reward, there was some skepticism on how much of this report is all novel breakthroughs, a la "did DeepSeek truly want Pipeline Parallelism" or "HPC has been doing the sort of compute optimization ceaselessly (or also in TPU land)".
Now, abruptly, it’s like, "Oh, OpenAI has a hundred million users, and we'd like to construct Bard and Gemini to compete with them." That’s a completely totally different ballpark to be in. Since launch, we’ve additionally gotten confirmation of the ChatBotArena ranking that places them in the top 10 and over the likes of latest Gemini pro models, Grok 2, o1-mini, and many others. With solely 37B lively parameters, that is extremely appealing for many enterprise purposes. It’s their newest mixture of consultants (MoE) model educated on 14.8T tokens with 671B total and 37B lively parameters. DeepSeek-LLM-7B-Chat is an advanced language mannequin trained by DeepSeek, a subsidiary company of High-flyer quant, comprising 7 billion parameters. Step 2: Download the DeepSeek-LLM-7B-Chat model GGUF file. 3. Train an instruction-following model by SFT Base with 776K math issues and their software-use-built-in step-by-step solutions. Probably the most spectacular half of those results are all on evaluations thought of extraordinarily onerous - MATH 500 (which is a random 500 problems from the full test set), AIME 2024 (the tremendous laborious competition math issues), Codeforces (competition code as featured in o3), and SWE-bench Verified (OpenAI’s improved dataset split). This stage used 1 reward model, skilled on compiler suggestions (for coding) and floor-fact labels (for math).
Reviews