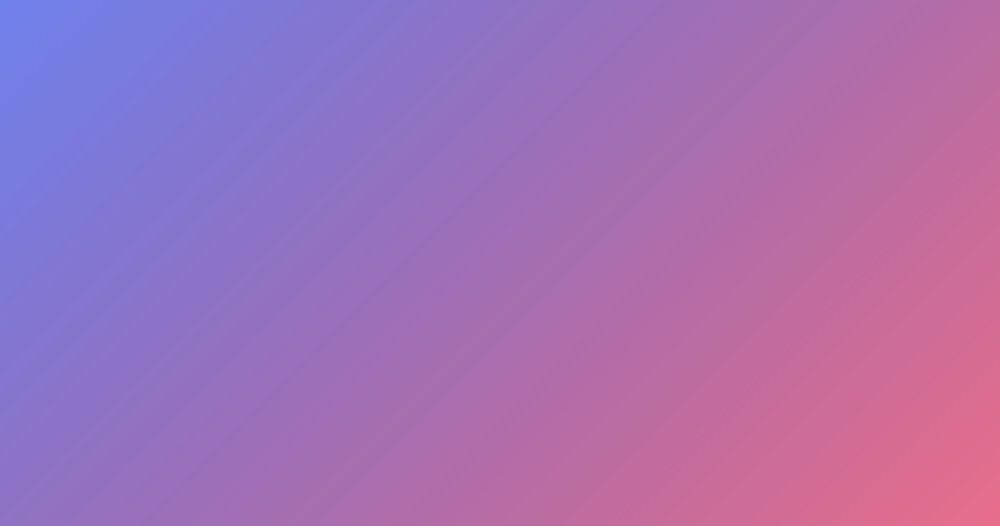
GitHub - Deepseek-ai/DeepSeek-V3
DeepSeek Coder. Released in November 2023, this is the corporate's first open source model designed specifically for coding-associated duties. Initial checks of R1, released on 20 January, present that its performance on certain tasks in chemistry, mathematics and coding is on a par with that of o1 - which wowed researchers when it was released by OpenAI in September. The model’s success could encourage more companies and researchers to contribute to open-source AI initiatives. Agree. My customers (telco) are asking for smaller models, much more centered on specific use circumstances, and distributed all through the community in smaller devices Superlarge, expensive and generic fashions should not that useful for the enterprise, even for chats. Be particular in your solutions, however train empathy in how you critique them - they are more fragile than us. The mannequin is open-sourced underneath a variation of the MIT License, permitting for industrial utilization with specific restrictions. The licensing restrictions replicate a rising consciousness of the potential misuse of AI applied sciences. Usage restrictions embrace prohibitions on military purposes, dangerous content material technology, and exploitation of weak teams.
In Table 2, we summarize the pipeline bubbles and memory usage throughout completely different PP strategies. DeepSeek reveals that lots of the modern AI pipeline shouldn't be magic - it’s consistent positive factors accumulated on cautious engineering and determination making. As for Deep Seek the training framework, we design the DualPipe algorithm for deepseek environment friendly pipeline parallelism, which has fewer pipeline bubbles and hides a lot of the communication during training through computation-communication overlap. DeepSeek, the start-up in Hangzhou that constructed the model, has released it as ‘open-weight’, meaning that researchers can examine and construct on the algorithm. The agency has also created mini ‘distilled’ versions of R1 to permit researchers with restricted computing power to play with the model. To hurry up the process, the researchers proved both the original statements and their negations. DeepSeek-V2.5 makes use of Multi-Head Latent Attention (MLA) to reduce KV cache and enhance inference pace. SGLang at the moment supports MLA optimizations, DP Attention, FP8 (W8A8), FP8 KV Cache, and Torch Compile, delivering state-of-the-artwork latency and throughput performance amongst open-supply frameworks.
DeepSeek-V3 stands as one of the best-performing open-source mannequin, and also exhibits competitive performance against frontier closed-source models. Firstly, DeepSeek-V3 pioneers an auxiliary-loss-free deepseek technique (Wang et al., 2024a) for load balancing, with the intention of minimizing the opposed impression on mannequin efficiency that arises from the hassle to encourage load balancing. Secondly, DeepSeek-V3 employs a multi-token prediction coaching objective, which we have observed to enhance the overall efficiency on analysis benchmarks. "Our work demonstrates that, with rigorous analysis mechanisms like Lean, it is feasible to synthesize large-scale, high-quality information. "We imagine formal theorem proving languages like Lean, which offer rigorous verification, characterize the future of mathematics," Xin mentioned, pointing to the rising trend in the mathematical neighborhood to use theorem provers to verify complex proofs. Future outlook and potential impression: DeepSeek-V2.5’s release could catalyze further developments within the open-source AI community and affect the broader AI business. Expert recognition and praise: The new model has obtained significant acclaim from trade professionals and AI observers for its efficiency and capabilities. Beyond the fundamental architecture, we implement two further methods to further improve the model capabilities.
Therefore, by way of architecture, DeepSeek-V3 nonetheless adopts Multi-head Latent Attention (MLA) (DeepSeek-AI, 2024c) for efficient inference and DeepSeekMoE (Dai et al., 2024) for cost-efficient training. DeepSeek has only actually gotten into mainstream discourse in the past few months, so I expect more analysis to go in the direction of replicating, validating and improving MLA. Recomputation of RMSNorm and MLA Up-Projection. This year we now have seen vital enhancements at the frontier in capabilities as well as a model new scaling paradigm. These two architectures have been validated in DeepSeek-V2 (DeepSeek-AI, 2024c), demonstrating their functionality to take care of sturdy model efficiency while attaining environment friendly coaching and inference. To run regionally, DeepSeek-V2.5 requires BF16 format setup with 80GB GPUs, with optimal efficiency achieved utilizing eight GPUs. Despite its excellent efficiency, DeepSeek-V3 requires solely 2.788M H800 GPU hours for its full coaching. The following coaching stages after pre-coaching require only 0.1M GPU hours. DeepSeek hasn’t released the complete cost of coaching R1, but it's charging people utilizing its interface round one-thirtieth of what o1 costs to run. However, in intervals of speedy innovation being first mover is a lure creating prices which might be dramatically greater and decreasing ROI dramatically.
If you loved this short article and you would like to receive more data with regards to ديب سيك kindly visit the webpage.
Reviews