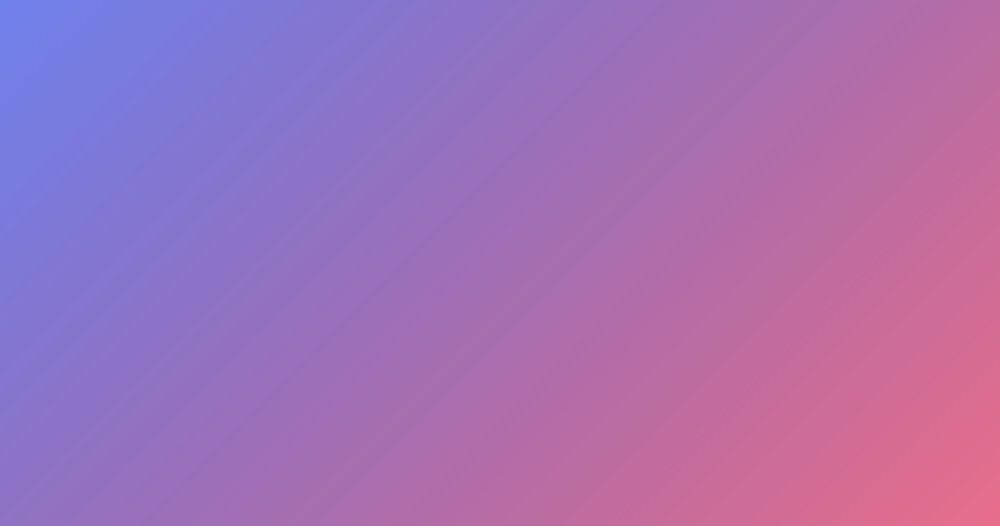
Deepseek Cash Experiment
Through intensive mapping of open, darknet, and deep seek internet sources, DeepSeek zooms in to hint their internet presence and ديب سيك establish behavioral purple flags, reveal criminal tendencies and actions, or some other conduct not in alignment with the organization’s values. There’s some controversy of DeepSeek coaching on outputs from OpenAI fashions, which is forbidden to "competitors" in OpenAI’s phrases of service, but this is now harder to prove with what number of outputs from ChatGPT are now typically accessible on the web. Chinese synthetic intelligence company DeepSeek disrupted Silicon Valley with the discharge of cheaply developed AI fashions that compete with flagship choices from OpenAI - but the ChatGPT maker suspects they were built upon OpenAI data. Anthropic, DeepSeek, and plenty of different companies (perhaps most notably OpenAI who released their o1-preview model in September) have found that this coaching greatly increases performance on sure choose, objectively measurable duties like math, coding competitions, and on reasoning that resembles these tasks. DeepSeek Coder. Released in November 2023, this is the company's first open source model designed particularly for coding-related tasks. The company's current LLM fashions are DeepSeek-V3 and DeepSeek-R1. Architecturally, the V2 fashions have been significantly modified from the DeepSeek LLM sequence.
The base model of DeepSeek-V3 is pretrained on a multilingual corpus with English and Chinese constituting the majority, so we evaluate its performance on a series of benchmarks primarily in English and Chinese, as well as on a multilingual benchmark. Compared with DeepSeek-V2, we optimize the pre-coaching corpus by enhancing the ratio of mathematical and programming samples, whereas expanding multilingual coverage beyond English and Chinese. As DeepSeek-V2, DeepSeek-V3 additionally employs additional RMSNorm layers after the compressed latent vectors, and multiplies extra scaling components on the width bottlenecks. In addition, in contrast with DeepSeek-V2, the new pretokenizer introduces tokens that combine punctuations and line breaks. As well as, we add a per-token KL penalty from the SFT mannequin at every token to mitigate overoptimization of the reward model. The reward for math issues was computed by comparing with the bottom-reality label. They recognized 25 varieties of verifiable instructions and constructed around 500 prompts, with each immediate containing one or more verifiable instructions.
Some of them gazed quietly, more solemn. People and AI systems unfolding on the page, becoming more actual, questioning themselves, deepseek describing the world as they noticed it and then, upon urging of their psychiatrist interlocutors, describing how they related to the world as nicely. So had been many different individuals who intently followed AI advances. "The most essential level of Land’s philosophy is the identity of capitalism and artificial intelligence: they're one and the same factor apprehended from completely different temporal vantage points. D is set to 1, i.e., moreover the exact next token, each token will predict one further token. 0.1. We set the utmost sequence size to 4K throughout pre-training, and pre-prepare DeepSeek-V3 on 14.8T tokens. The gradient clipping norm is set to 1.0. We make use of a batch measurement scheduling technique, the place the batch dimension is progressively increased from 3072 to 15360 within the training of the first 469B tokens, after which retains 15360 within the remaining training.
In the present process, we need to read 128 BF16 activation values (the output of the previous computation) from HBM (High Bandwidth Memory) for quantization, and the quantized FP8 values are then written again to HBM, solely to be learn again for MMA. Throughout the backward cross, the matrix needs to be read out, dequantized, transposed, re-quantized into 128x1 tiles, and stored in HBM. In our workflow, activations during the forward go are quantized into 1x128 FP8 tiles and saved. To handle this inefficiency, we recommend that future chips combine FP8 cast and TMA (Tensor Memory Accelerator) access right into a single fused operation, so quantization might be completed in the course of the switch of activations from international reminiscence to shared reminiscence, avoiding frequent memory reads and writes. Combined with the fusion of FP8 format conversion and TMA access, this enhancement will considerably streamline the quantization workflow. Support for Online Quantization. Current GPUs only assist per-tensor quantization, lacking the native help for high-quality-grained quantization like our tile- and block-wise quantization. The present architecture makes it cumbersome to fuse matrix transposition with GEMM operations. Support for Transposed GEMM Operations. The current implementations battle to successfully assist on-line quantization, regardless of its effectiveness demonstrated in our analysis.
If you loved this report and you would like to receive a lot more data relating to ديب سيك kindly take a look at our own web-site.
Reviews